Rough Correlation for Tail Dependence in Asset Price Pair Models
Research Seminar by Dr. Márkus László – Institute of Finance.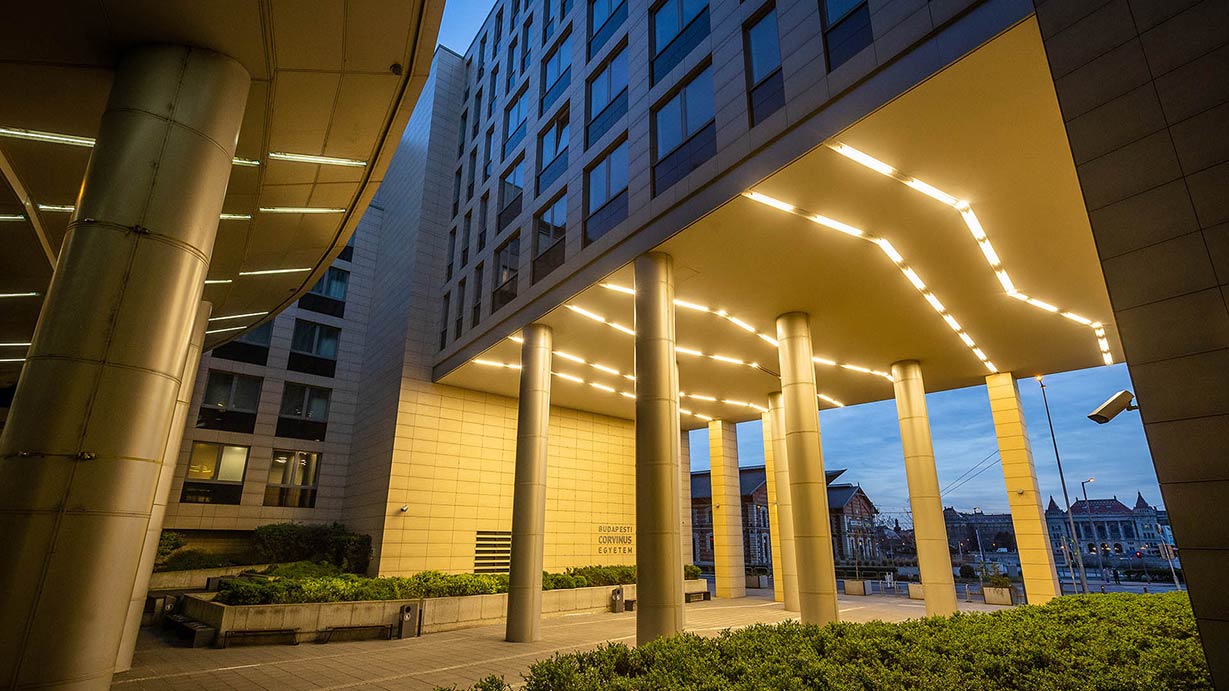
Date: 25 September, 2023, Monday, 12.00-13.00 (Budapest time)
Venue: Corvinus Main Building, Auditorium E.III. (ground floor)
Language: English
Dr. Márkus László: Rough Correlation for Tail Dependence in Asset Price Pair Models
Abstract: A cover story of the popular magazine ’Wired,’ published in 2009, carried the title ’Recipe for Disaster: The Formula That Killed Wall Street’. It tried to blame the then-actual financial crisis on the mathematics used in computing default probabilities. The selected scapegoat was the Gaussian copula, the contemporary industry standard in finance to describe the association structure of a pool of loans, bonds, or assets. The Gaussian copula cannot create tail dependence, crucial in modeling simultaneous defaults, but that was known before the crisis, as were other models capable of doing so. Almost 15 years passed since then, but the various copula and other models, going beyond correlation for describing dependence, do not harmonize well with the stochastic differential equation (SDE) description used routinely for individual financial instruments.
In the lecture, I reconsider the static, single-number Pearson correlation for describing probabilistic dependence and build up an approach where interdependence is timedependent and random, so a stochastic process describes it. This process is inherent in the quadratic covariation for Brownian motions – the driving forces in the asset price equations. These covariations, in turn, are integrals of stochastic processes, called stochastic correlations, driven by SDEs. The goodness of the suggested model is tested on historical asset price data using Kendall functions of copulas.
The paradigm of rough paths leads to a newly emerging methodology in modeling the stochastic volatility of assets. I suggest a similar approach to the mentioned stochastic correlations. I show that in frequent, minute-wise trade, temporally localized correlations have the fractal property. The corresponding fractal dimensions, as well as the Hurst exponents, support the assumption of rough paths. The developed model helps show that brokers’ herding behavior, as expressed by the HIX index, may lead to very different tail dependence and, hence, variable probabilities of coincident defaults.
Classical statistical tools have proven to be computationally intensive and, therefore, slow for identifying the correlation process either by estimating it from historical data or calibrating the model to option prices. That invokes the use of AI tools for estimation, and indeed, higher accuracy can be achieved much faster by effectively training a proper neural network.
More information on future research seminars is available here.